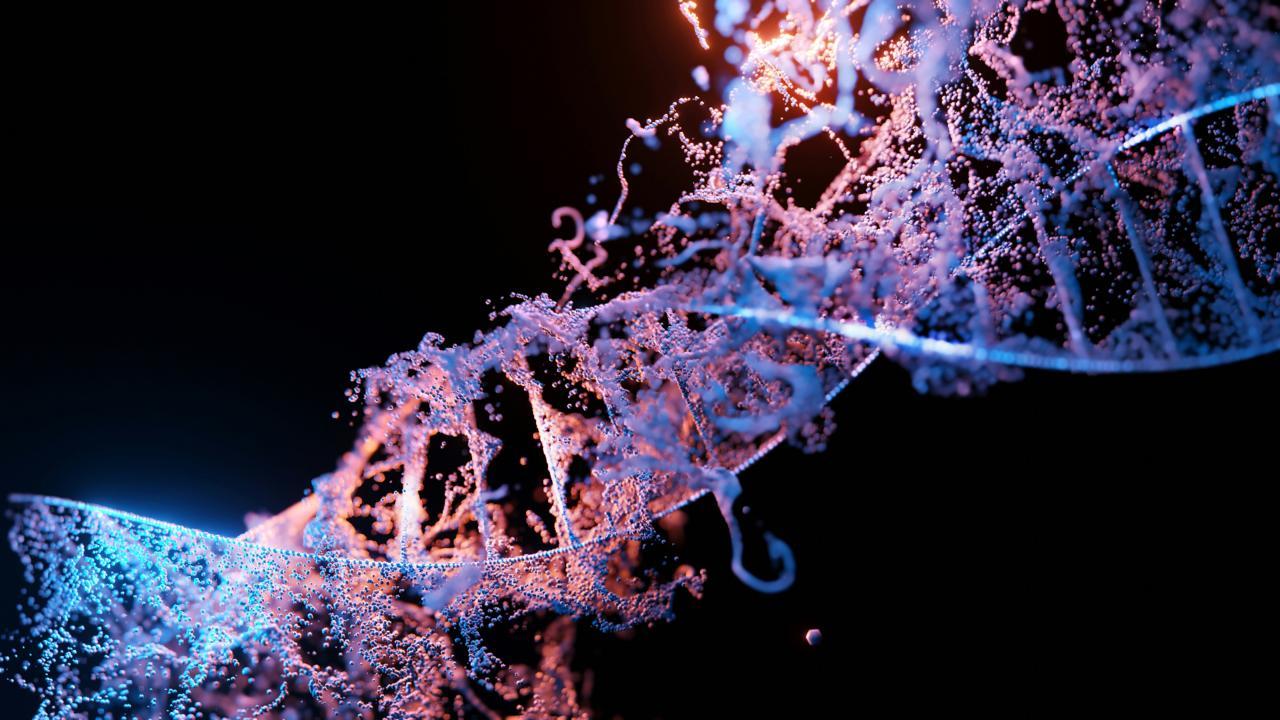
How Mathematics Informs Molecular Biology
For many, mathematics exists solely within the confines of a blackboard, a calculator or a textbook. But ask Javier Arsuaga and he’ll tell you that mathematics exists within us, right down to our DNA.
“Biology is very, very complicated and different areas of math can be applied to solve different types of problems in molecular biology,” said Arsuaga, a professor with joint appointments in the Department of Mathematics and the Department of Molecular and Cellular Biology. “Can we develop mathematical models that make biology more predictive? That’s where the richness of mathematics comes into play.”
The study of our ever-shifting, tangle of a genome is called topology. Arsuaga harnesses this area of mathematics in tandem with machine learning and computational modeling to investigate how diseases, like breast cancer, spread.
“You’re allowed to stretch DNA, you’re allowed to fold it, you’re allowed to coil it as much as you want, but you’re not allowed to break it,” said Arsuaga. “In humans, disruption of the 3D structure of the genome is the signature of DNA-damaging agents and has been associated with a wide range of diseases, including cancer.”
Applications of the abstract
Arsuaga’s fascination with the intersection of mathematics and biology traces back to his days at the University of Zaragoza in Spain.
As a mathematics major, he was entranced by the abstract nature of the subject but as he came closer to pursuing a doctoral degree, his tune changed. He learned about the work of De Witt Sumners, a distinguished professor of mathematics and a member of the Institute of Molecular Biophysics at Florida State University. Sumner was pioneering the application of knot theory—the study of closed, three-dimensional curves and their deformations—to better understand the structure of DNA.
“That changed the trajectory of my career because it really sparked my interest in molecular biology and its connections to abstract math,” Arsuaga recalled.
With a course of study identified, Arsuaga obtained a Ph.D. in mathematics from Florida State University. All the while, he conducted research delving into the nuances of DNA packaging in bacterial viruses and developing wet lab techniques to improve the resolution of knotted DNA molecules, among other projects.
After completing his doctoral degree, Arsuaga spent five years conducting postdoctoral work at UC Berkeley and the University of California, San Francisco’s Helen Diller Family Comprehensive Cancer Center. During this time, he learned about chromosomal aberrations, which are morphological or numerical alterations that can lead to cancer.
The work laid the foundation for Arsuaga’s cancer research at UC Davis.
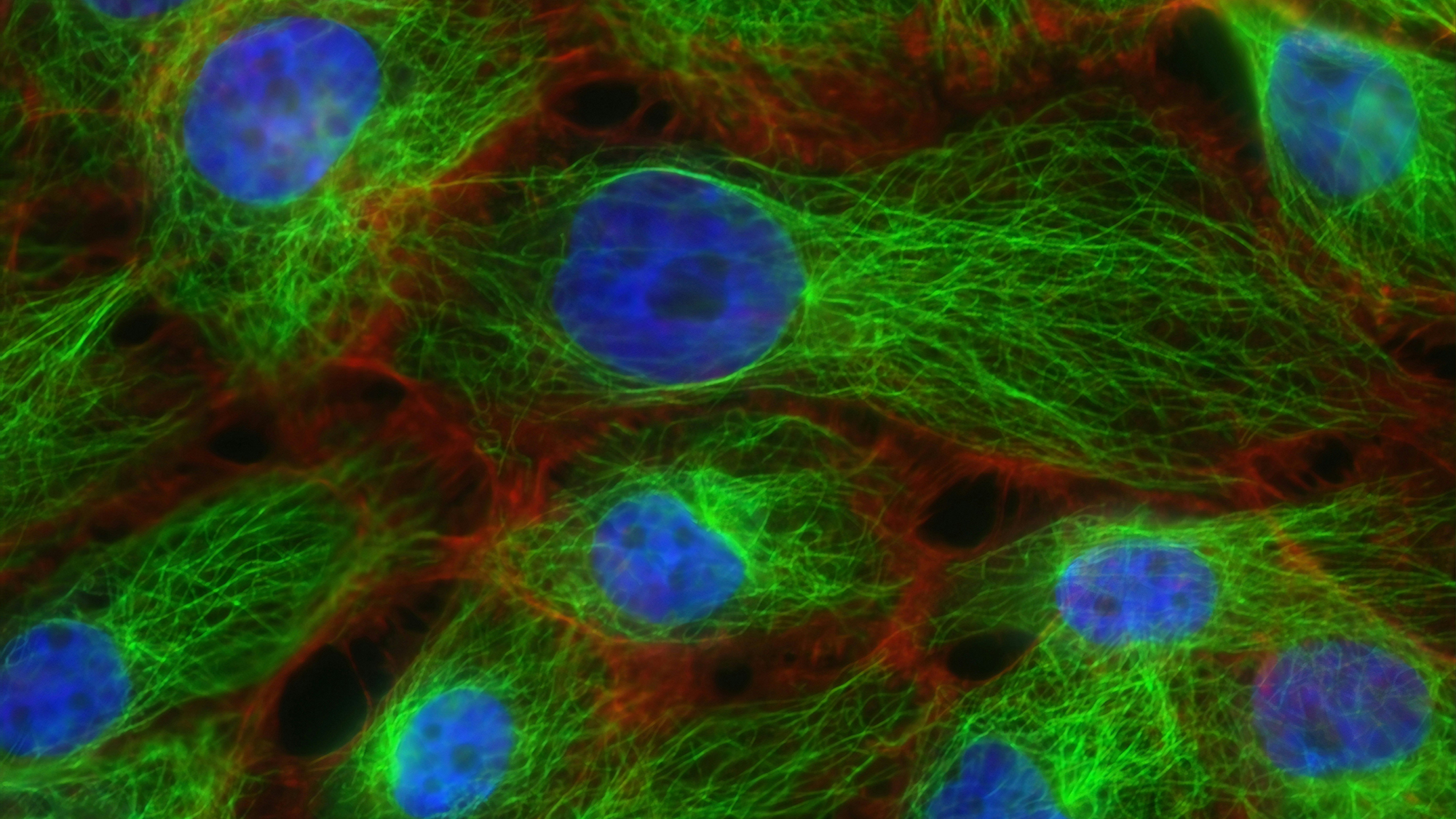
Identifying cancerous genes
At UC Davis, Arsuaga and his colleagues use topological data analysis to identify the genes that lead to the development of different types of breast cancer.
“There are four main subtypes of breast cancer: luminal A, luminal B, basal-like and HER 2,” Arsuaga explained. “Patients with different subtypes receive different treatments, so they are, in effect, different diseases.”
While treatments vary, the underlying causes of these four subtypes of breast cancer are similar. They’re diseases characterized by uncontrolled cell growth, which is generally regulated by different oncogenes and tumor suppressor genes.
“The oncogenes are like the accelerator of a car. It makes the cell cycle advance,” Arsuaga said. “And the tumor suppressor genes are like a brake that prevents the cell cycle from continuing.”
Chromosomal aberrations make this process go haywire, leading to a competitive cellular environment in which oncogenes are amplified and tumor suppressor genes are deleted. In the end, this genetic interplay promotes tumor growth.
“We’ve refined the theory of topological data analysis to identify these amplifications and deletions across the genome for different cancer subtypes to hopefully identify genetic information that other research groups may have missed,” Arsuaga said. “Once you detect these amplifications and deletions, you can dig deeper into that region of the genome and see what genes are in that particular region.”
By studying the genomes of multiple breast cancer patients, Arsuaga and his colleagues hope to identify what genes lead to the development of different breast cancers. If common genes are identified across patients with a specific breast cancer subtype, the hope is that the information could lead to better treatments.
Such large datasets aren’t just informing Arsuaga’s research into the causes of breast cancer. It’s also informing his work to prevent future pandemics.
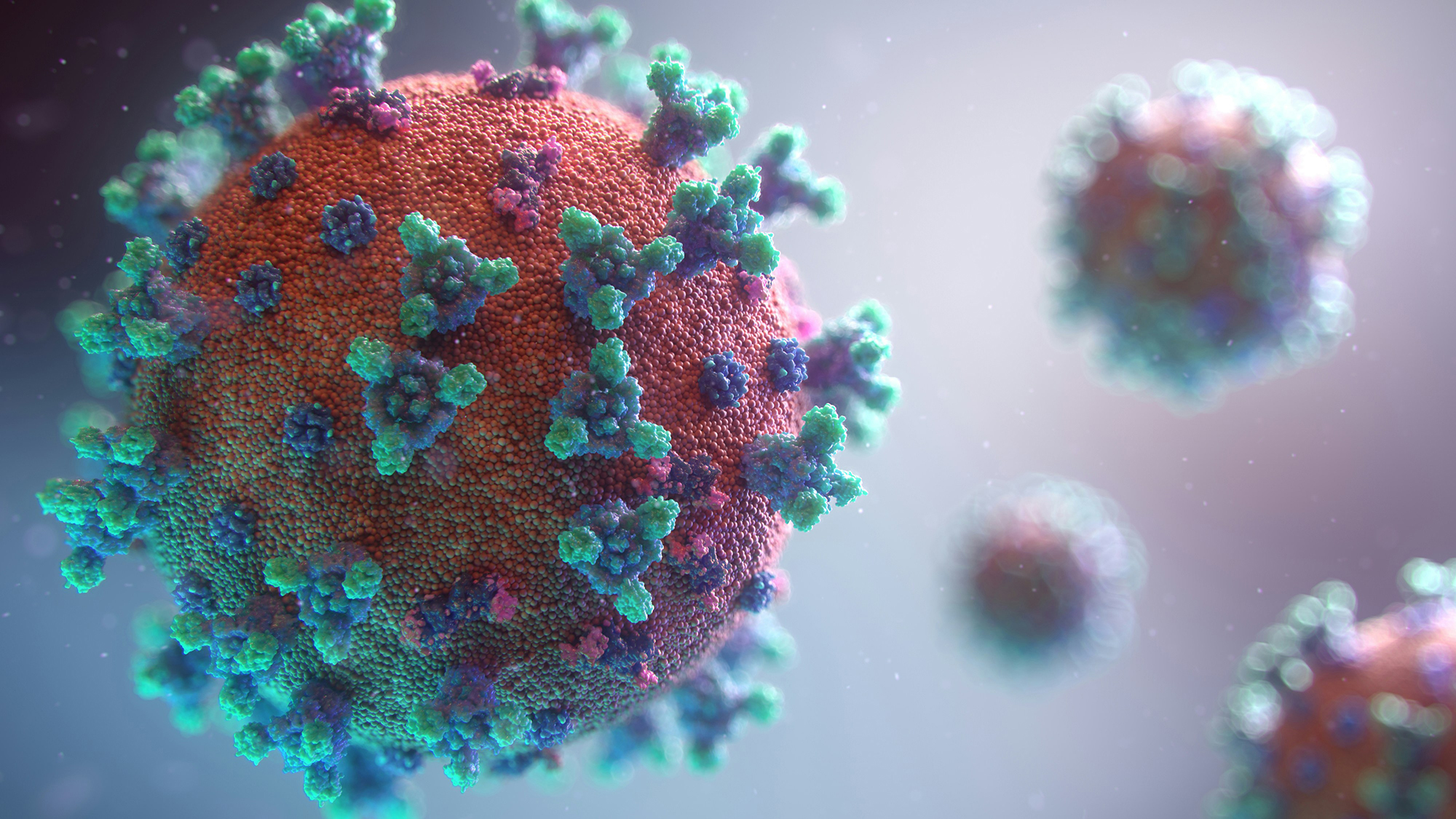
The coronavirus mutational landscape
During the COVID-19 pandemic, researchers were on the frontlines of mitigating the spread of the SARS-CoV2 virus. In the wake of the pandemic, Arsuaga and an interdisciplinary team of researchers developed an artificial intelligence model capable of identifying coronaviruses that could “spill over” from animals to humans.
When a coronavirus sequence is fed into a deep learning model, the model produces a human binding potential, or the probability of the virus spike protein’s ability to bind to human cells.
“We identified three different viruses that were unknown to bind to human receptors,” Arsuaga said.
The team then ran molecular dynamics simulations that elucidated how such binding could occur. Arsuaga is now working with Priya Shah, an assistant professor in the UC Davis Department of Chemical Engineering and Department of Microbiology and Molecular Genetics, to conduct experimental lab work that confirms what they’ve seen on the theoretical side. Such research could help prevent or mitigate future pandemics by allowing researchers to develop vaccines before a virus spreads.
“That’s the power of machine learning,” Arsuaga said. “We’re experiencing a revolution at the level of data and data generation, computer science and algorithm generation.”
Media Resources
- ARSUAGA VAZQUEZ LAB - Molecular Biology Lab, UCDavis
- Greg Watry is a content strategist and writer in the College of Letters and Science.